▶ Representation learning
Machine learning exists everywhere. In the field of medical image processing, representation learning provides novel insights for understanding the data. Representation learning is a machine learning approach focused on automatically discovering meaningful features or representations from raw data. By learning abstract representations, representation learning facilitates improved performance across diverse tasks like classification, regression, clustering, and generative modeling, etc.
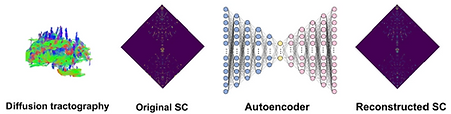
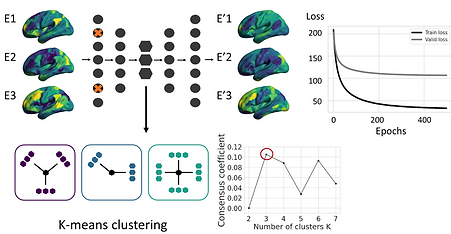
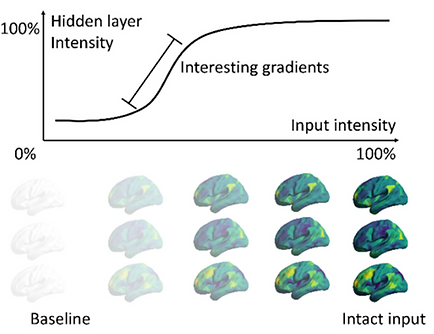
▶ Generative model
Generative models learn the distribution of training images and generate new images that exhibit similar characteristics. There are several types of generative models, such as Generative Adversarial Networks (GANs), Variational Autoencoders (VAEs), and diffusion models etc. These approaches can be used in tasks like image synthesis, style transfer, and data augmentation.
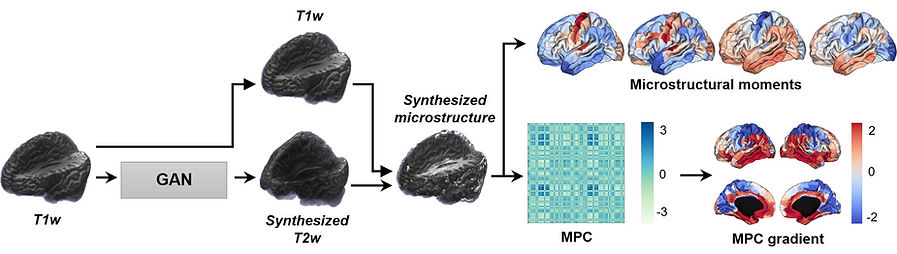
Park et al., NeuroImage, 2024.
▶ Segmentation & Parcellation
Segmenting small brain lesions is difficult compared to large lesions. To reduce false positives, feature extraction, advanced feature selection, and classification approaches can be used.
Parcellation is one of the major issues in explaining brain regions or networks. Various ways are used to define brain regions/networks, such as ICA and clustering techniques. Connectivity-based parcellation (CBP) is a widely used approach to dividing brain regions into functionally distinct subregions.
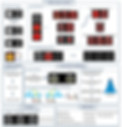
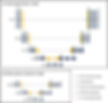

